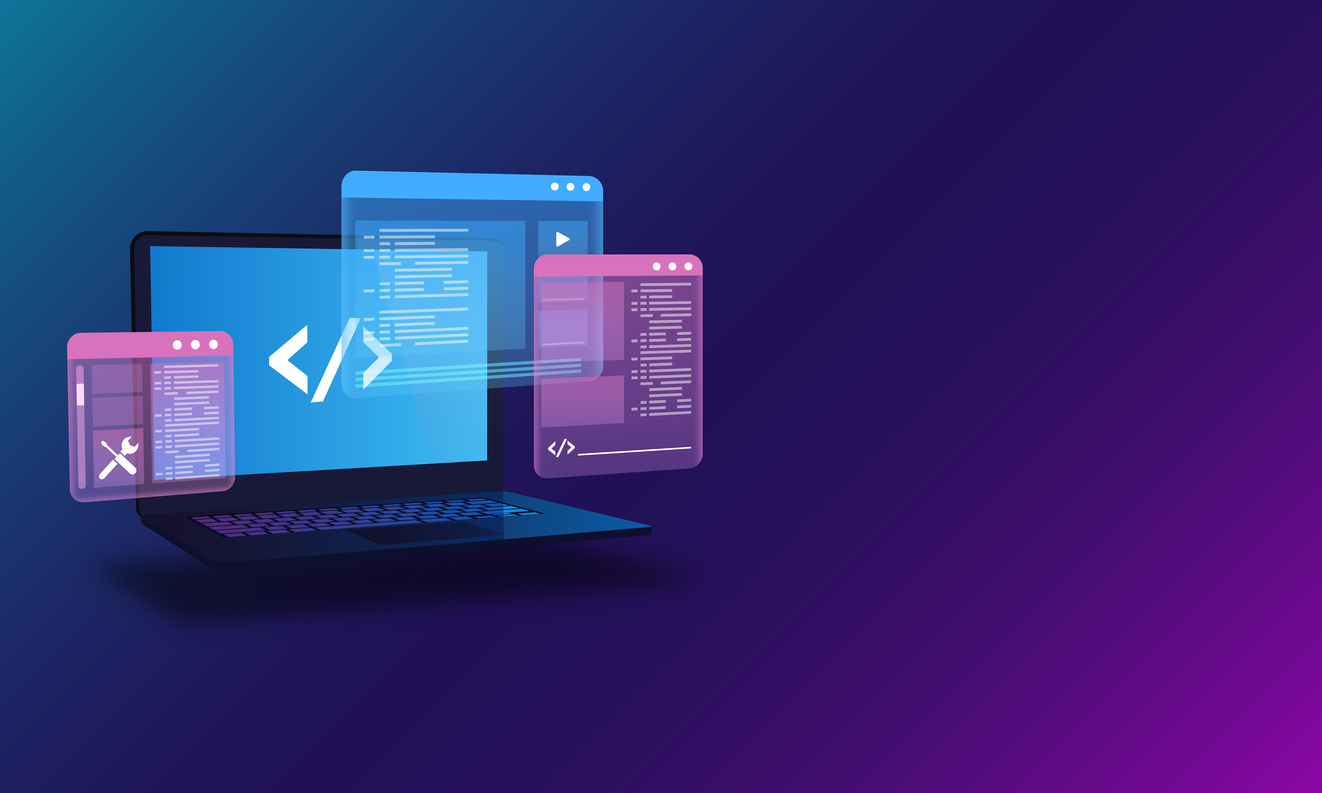
Introduction
AI is not just chatGPT. Artificial Intelligence (AI) is a field that uses computers to make lots of calculations. In other words, it’s like having 100 math nerds at your fingertips. (There’s no magic, just tons of math.) ChatGPT is an example of AI that has been popularized as of late and is fairly easy to access. But “easy to access” doesn’t mean that it’s the best AI tool for what you want to accomplish with your business.
AI is a science – one that requires experimentation (which can be expensive if you don’t have clearly defined goals). If you haven’t identified the top roadblocks in your business/industry (or if you don’t understand how they came into being or their impact), you may not be ready for AI just yet. AI can tell you things like possible customer decision outcomes, possible staff decision outcomes potential areas of improvement In short, AI can help you make better decisions – but it can’t make the changes to your business for you. It can lead you to the well, but making the decision to drink? Totally up to you.
In this series, we’ll look at areas where AI may be able to help – starting with how AI can help increase customer retention.
CHAPTER 1:
How to decrease customer “churn” (attrition) using AI
In AI we often talk about being able to predict customers’ actions. But that’s not actually what we mean. You see there’s usually a pattern of actions that lead up to a desired action. We predict the likelihood that a customer will complete that desired action. We train our models to be as accurate as possible using customer data points.
Back in 2012, Target got in trouble for this. They had a predictive model that could predict whether or not a customer was pregnant based on their recent purchase history. Their AI shifted through customer data points, and identified behaviors that lead to purchasing pregnancy and baby products.
They would then mail coupon books in hopes of getting the customer to purchase from them. The problem? They sent a book to a teen. The teen’s parents got mad and went to the store to complain. Turns out the parents were wrong.
See full article: https://techland.time.com/2012/02/17/how-target-knew-a-high-school-girl-was-pregnant-before-her-parents/
This is powerful math. And that was over 10 years ago. Target has since decided to bundle coupons to prevent theses conflicts, but the technology has advanced over time. See models are usually more affordable to implement than ChatGPT and more effective overall.
What is Predictive Analytics?
Predictive analytics is a sub-field of AI, focused on determining possible future outcomes. It can answer questions such as:
- Which customers are likely to churn?
- Which are likely to return items?
- Will my patient have an adverse episode?
- What will the company’s revenue look
- like in the next fiscal year?
- Which transactions are likely to be fraudulent?
- What personalized offers should be made to individual customers?
There are other areas such as
- Descriptive Analytics – What has happened. Basically, those dashboards and spreadsheets you hate to look at. They are describing what has already happened.
- Prescriptive Analytics – What you might need to do. Like those recommendations for purchases you see on Amazon. We’ll get more into this later.
Predictive Analytics involves creating a statistical model to run several simulations. Those simulations are usually averaged to give you a probability score. These probabilities can represent positive or negative actions.
That’s why you want to ask the right questions. That’s the difference between creating an AI project that succeeds and one that fails. It’s what leads to the three (3) biggest mistakes businesses make.
The three biggest mistakes businesses make
Ask the right questions so that you don’t have an “uh oh” moment.
1. The Data is not available
This next section is going to hurt a few feelings but it’s my book so I’m saying it. You may have sent a lot of money already, but you may not be collecting the data that you need. It’s not uncommon for me to walk into some consulting work to find that the company wants to do something, but the data was never collected. It’s also not uncommon for the data to be misformatted.
You need to know what data you have and how it is formatted. Artificial Intelligence uses mathematics to review patterns in the data that it receives. Fails happen because the data isn’t present, or it is present but it’s not representative of your current customers.
For example, I was listening to Wingstop CEO talk about how they’ve gone from serving lower-income to more middle-income single individuals. These customer behaviors ‘drift’ over time. They could even look completely different.
The models you create to represent user behaviors need to reflect this. These models work because the data is representative of the patterns — to build the right solution for you, it needs to have the right information.
Unless you have a data engineering team, a data scientist should not be your first hire. Even then, you really want to be sure to hire a data architect, even temporarily, who can ensure your data infrastructure is being designed for scalability and usability. Oh, and regulations if your industry has them. We’ll release a separate white paper on this but without this, you’re dooming your AI projects. It’s like sending a scientist to a room without lab supplies. Invest in quality supplies, not fancy shiny ones and get more out of your experiments.
Lack of data availability kills many data science projects every year.
2. Not aligning with the business KPI
What are your business goals? And do your data science teams know what they are? Having a data science team, and using a data science team effectively are two different things. Don’t build your AI projects in a silo – it leads to an 85% failure rate in AI initiatives. I like to think of myself as a mad scientist working on data experiments in a lab I made up in my mind. I can literally build anything and without direction at work, I could build something completely useless to the business.
Projects aligned with the desired business outcome require conversations with all departments that would benefit from the implementation. This could take 1-2 months of hammering out objectives, impact, measurement tactics, and cost all done before the large data science team is given access. You need an AI champion that knows AI and the business to make connections to the work and the bottom line.
As far as metrics are concerned, there’s so much more to it than I could cover in any simple book or whitepaper. I’ll only give a quick overview and hope that you hire the right statistician to get you to the next level. Here’s a quick overview of the ones I think you should be sure everyone knows. :
- Success metrics – what does the business care about? What does the business want? It should be put into words and then into metrics.
- Guardrail metrics – What things need to stay the same throughout? These are meant to protect the trustworthiness of the experiments. This relates to ensuring that infrastructure and data processing remain Sometimes projects don’t fail related to the experiment but latency, population size, other things.
- Driver metrics – shorter-term, faster-moving metrics that let you know everything is on track to reach the longer-term success metric. What does it take for the org to succeed? Aligning the overall AI strategy and subsequent experiments to driver metrics helps keep everyone accountable to the success metric.
This list isn’t exhaustive. But it does include the high-level considerations.
At the back of the book, there’s a checklist to help you better ask questions to get to these metrics.
3. There are different types of AI.
This one is more of a bet peeve but it’s my book so here we go.
Ignore your sales guy. Simple models have the potential for large impacts, require less time to implement, and cost less to get off the ground – but they are much less “fun” for data scientists and don’t require large labor teams from consultancy. They may decide to use a more complicated tool because it’s interesting or would make work more complex.
Having an AI champion will help you not choose the hype but the best solution. Sometimes, stakeholders will hear about a certain type of model in the news and request the team to use it. Honestly not having an AI champion should be a mistake but I’m only doing three. I’ve seen some pretty big mistakes happen from this. Ask for the options, check on the cost of implementation, and then choose the right approach. Hype is not the answer.
Chapter 2:
What types of predictive models should you be using?
There’s usually going to be more than one thing that you can do. Usually. That’s true with how you use math to approach your business problems. What the team chooses needs to be based on business objectives, cost, and capabilities.
But it all starts with asking the right questions.
What do you ask?
- Do you know the specific behavior you want to predict?
- Do you have access to a lot of needed historical data?
- Does it include all the information needed for what you wish to do?
- Do you need to understand how all variables relate to each other?
- Do you have an intervention in mind that you want to consider?
And remember, identify the metrics that will help you provide that your AI intervention is working.
Top model types for working with customer behavior:
- Next Purchase: How likely the desired customer (based on past purchase behavior) is to purchase from you in a certain time period (e.g., month, quarter)
- Response: How likely the desired customer (based on past purchase behavior) is to respond positively/negatively to marketing messaging.
- Next Best Offer: How likely the desired customer (based on past purchase behavior) is to take advantage of this offer.
- Binary Outcome Prediction: How likely the desired customer (based on past purchase behavior) is to make a purchase from you in a certain time-period (e.g., month, quarter)
- Uplift: How likely the desired customer (based on past purchase behavior) is to respond positively to specific interventions.
There are different methodologies to implementing these models. I’ll leave that up to your data science team. You’re responsible for ensure you understand if it’s working or not and holding them accountable to that.
Now, the output of these models are scores or classifications between categories. How you use the scores is a part of how useful AI will be in your business. If your team isn’t collaborative across how the output will be used. You will not get the results that you want.
Here’s some ways you can put them to use.
How to use this information
Sales Drivers
While you may easily see where a sale occurred, it doesn’t mean you automatically understand why the sale happened. Techniques used to build predictive models can help you improve the sales process.
Personalized Discounts
There are customers who buy at full price, who buy at a discount, and who won’t buy at all. AI helps you give the right discount offers to the right customers (and do it live on your website too).
Customer Journey Segmentation
Some customers may need alternative experiences with your product or service. Segmenting the output helps you design just the right paths or experiences for your customers.
Special Offers
Limited Time Offers (or offers for a certain customer segment) shouldn’t be sent to all customers – nor should they be left up to chance. Segmenting specific customers to send them offers tailored to their needs can help increase returns.
Examples of Applications that use similar techniques:
Disease
Thanks to doctors meticulously labeling data, computers can now predict if you have an illness from your medical imaging (and hopefully improve early disease detection).
Bank Fraud
While it may seem creepy, banks track all of your transactions in order to identify fraud. When something is done out of the norm, they are quick to send a message to confirm it‘s you.
Warehouse
Every wonder why platforms like Amazon never seem to run out of stock? They use predictive AI algorithms to monitor warehouse supply and restock, so you rarely have to wait for it to come back into stock.
Loyalty programs
Companies have loyalty programs for a reason. When you sign up, you give them tons of data – and they use that data to send you customized offers you’re more likely to take action on. From special “increased miles offer” from credit card companies to those extra toppings or drinks, it’s usually predictive technologies at play.
Discount offers from ecommerce brands
You’ve probably been shopping on a website when you get a pop-up (or receive an email) with a discount. That discount/offer is usually carefully crafted for your buying persona, and maximizes the chances you’ll buy everything in the cart.
Case Studies
Financial Client Churn Detection using Computer Vision
It is 5 – 25 times more expensive to acquire a new customer than to retain an existing one. While there are not many customers in financial services that churn (about 5-7% a year), the impact of their churn is larger due to the size of the investments. While regulations have slowed the process of implementing AI across organizations, personalized offerings still increase customer retention.
See the full video here: https://youtu.be/igT8G_-43Sc?si=gpSy_HWmU7T7syaO
Reasons for churn in wealth management:
- 89% leave due to poor customer service
- 59% of customers ages 25-35 will report their departure online
- Poor offering and pricing leads to offering the wrong products
- Life events such a marriage and job loss
Besides customer data and product data, they include market data. The data is highly imbalanced (i.e. number of customers in each category. 95% who don’t churn, 5% who do.) since they have a low churn rate.
Additionally, they do not split the data in the traditional methodology. They split the data over time since there may not be regular transactions.
They used computer vision to read documents.
Using ML for Customer Retention by AI Product Managers
AI PM Community Session #47
With a mix of predictive analytics and GenAI, you can create a solution to help increase customer retention. (It requires a good amount of data governance to make it work.) It starts by predicting if an account will churn. Next, they use an AI “copilot” to help summarize the account without going to the account manager. (Data governance enables the right data to be available.)
See full video here: https://youtu.be/O-g9n_G3lAQ?si=iRMDgekQhDzqB-_d
KPIs for this type of solution:
- Customer churn rate
- Average revenue per user
- Customer lifetime value
- Customer satisfaction score
- Repeat purchase rate
- Up-selling additional products
Cost considerations:
- Cost of data
- Cost of models
- Cost of modifying/creating an application
- Cost of integrating workflows
- Cost of consumption of API
- Human cost (training, implementation, R&D)
- Cost of maintaining infrastructure
Grow Customer Revenue with Predictive AI
Gartner TGI Conference
The biggest roadblocks to streamlined customer engagement are a lack of collaboration across revenue functions and deficient customer data. AI needs to understand the full buyer situation. A product-centric approach that doesn’t involve the business means a less engaged experience.
See the full video here: https://youtu.be/igT8G_-43Sc?si=gpSy_HWmU7T7syaO
Blockers to effective customer engagement:
- Siloed teams
- Fragmented data
- Time to build comprehensive
- data solutions
Conversational Intelligence can help
- identify buying opportunities:
- call analytics
- social listening
- voice assistants
- CRM and sales enablement platforms
- Chatbots
- Market Research
Effective collaboration across teams helps create consistent messaging for customers. By layering conversational intelligence techniques on top of propensity scoring, lead/account scoring, and customer segmentation techniques, you improve your confidence in using predictive analytics for customer expansion.
Chapter 3:
Five ways to fix those mistakes – and fast
Audit your data
With the amount of data being created at your company, AI will one day be able to tell you which KPIs you need to track, the impact of making changes, and what strategies you need to consider – all on a friendly dashboard. You need to know what data is available to you. I’ve walked into many situations where the decision maker didn’t know the data they had access to in their databases. Some thought I was making it up, while others wanted to try to work around it because they didn’t have the right data available. In both situations, you don’t really get what you want.
Make sure to implement data governance principles to know what’s available, when it’s collected, and how it’s used. A general overview for the top stakeholders will help enable better decision-making – which a data catalog will help you manage.
Know what goes into the one KPI you want to change
Understanding the one metric that the business wants to improve will help you better determine how to use AI in your business. To understand it, you need to know the processes, the data, and the stakeholders to get a full understanding of the problem. Only then can you determine the proper options and solutions for implementations.
The Future of the Small Business Data Layer
With the amount of data being created at your company, AI will one day be able to tell you which KPIs you need to track, the impact of making changes, and what strategies you need to consider – all on a friendly dashboard.
Be realistic about the cost
That brings me to this point: It always costs more than you think it will. While AI implementations are made with code, they run on servers and inside of networks – all of which cost money. The cost of deployment can pause (or even at times, stop) work. To understand the cost, you need to build an architecture for running it for the end user. This helps the data scientist build the model. If they understand the constraints, they are better able to stay within budgetary requirements
Upskilling Impacted workers.
Sabotage has destroyed more than one AI project. The workforce is scared of losing their positions to Ai, and companies have no clear messaging on how their work will change.
There’s a sense of loss when AI takes over a job, whether or not the employee hated the work. Companies implementing AI solutions may need to spend time on change management, being prepared with messaging that resonates with the workers, and which explains their plan for upskilling the employees impacted by the AI implementation. Upskilling (when implemented correctly) helps improves company morale, strengthens the company culture and helps build more loyalty.
Appointing an AI lead
AI implementations require a leader – someone who has been with the company for a while, and who you can trust to understand AI fundamentals. (This is the most important fix you can make.)
Whether you build an in-house team or outsource the role, an AI leader makes all the difference. Without trust, it’s hard to build anything meaningful. Without the ability to experiment, it’s hard to be successful. When businesses lack this, salespeople may promise the world to you, and then blame the data scientists for not delivering – even though the data scientist was never brought into the conversation, and the in-house team never knew what was truly needed.
References
- Info World – “Why AI investments fail to deliver”
https://www.infoworld.com/article/3639028/why-ai-investments-fail-to-
deliver.html
- Segment – “Customer Retention Model: Definition, Creation & FAQs
https://segment.com/growth-center/customer-retention/models/
- User Pilot – How to Build a Churn Prediction Model to Predict Customer Churn
https://userpilot.com/blog/churn-prediction/